
Hyperplane separation theorem - Let A is closed polytope then such a separation exists. In the context of support-vector machines, the optimally separating hyperplane or maximum-margin hyperplane is a hyperplane which separates two convex hulls of points and is equidistant from the two. The Hahn–Banach separation theorem generalizes the result to topological vector spaces.Ī related result is the supporting hyperplane theorem. The hyperplane separation theorem is due to Hermann Minkowski. An axis which is orthogonal to a separating hyperplane is a separating axis, because the orthogonal projections of the convex bodies onto the axis are disjoint.
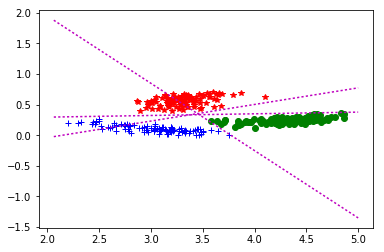
In another version, if both disjoint convex sets are open, then there is a hyperplane in between them, but not necessarily any gap. In one version of the theorem, if both these sets are closed and at least one of them is compact, then there is a hyperplane in between them and even two parallel hyperplanes in between them separated by a gap. There are several rather similar versions.
#Hyperplan separateur labels how to#
I'm not sure how to plot a number line, but you can always resort to a scatter plot with all y coordinates set to 0.In geometry, the hyperplane separation theorem is a theorem about disjoint convex sets in n-dimensional Euclidean space. Draw a random test point You can click inside the plot to add points and see how the hyperplane changes (use the mouse wheel to change the label). This hyperplane could be found from these 3 points only. So to visualize, you merely need to plot your data on a number line (use different colours for the classes), and then plot the boundary obtained by dividing the negative intercept by the slope. The optimal separating hyperplane has been found with a margin of 2.00 and 3 support vectors. That's your 0-d hyperplane (point) for the classifier. Now try changing the means of the distributions that make up X, and you will find that the answer is always -intercept/slope.

Ici b est utilis pour slectionner l’hyperplan c’est-dire perpendiculaire au vecteur. Ceux-ci sont communment appels vecteurs de poids dans l’apprentissage automatique. However, if you take y=0 and back-calculate x, it will be pretty close to 5. Un hyperplan sparateur peut tre dfini par deux termes : un terme d’interception appel b et un vecteur normal d’hyperplan de dcision appel w. Once you fit the classifier, you find out the intercept is about -0.96 which is nowhere near where the 0-d hyperplane (i.e. Intuitively it's clear the hyperplane should be halfway between 0 and 10. y is the array of labels (100 of class '0' and 100 of class '1'). After that though I went to get the relative distances from the hyper-plane for data from each. After fitting the data using the gridSearchCV I get a classification score of about. X is the array of samples such that the first 100 points are sampled from N(0,0.1), and the next 100 points are sampled from N(10,0.1). I'm currently using svc to separate two classes of data (the features below are named data and the labels are condition).

#Hyperplan separateur labels code#
So I decided to play with the sample code below to see if I can figure out the answer: from sklearn import svm I've spent about an hour looking for answers in the documentation for scikit-learn, but there is simply nothing on 1-d SVM classifiers (probably because they are not practical). So the question is really how to turn this line into a point. Yet what scikit-learn gives you is a line.

On the surface it's very simple - one feature means one dimension, hence the hyperplane has to be 0-dimensional, i.e. The authors observed that the survivability requirements increase the problem size dramatically and that in this case, the cutting plane algorithm only slightly improves the LP relaxation lower.
